The investment industry constantly changes, with new technologies and strategies always emerging. One of the most exciting developments in recent years is machine learning (ML). ML makes it possible to identify high-potential stocks more accurately by leveraging powerful algorithms and vast amounts of data.
What is machine learning, and how is it related to mutual funds and stocks?
Machine Learning is a sector of artificial intelligence based on the idea that a computer program can teach itself new information and adjust its behavior in response to it without direct human involvement. Many trading platforms, such as metatrader 5, support using ML as it helps investors uncover new investment possibilities.
Buying stocks means getting a share of a particular corporation, while a mutual fund is a vehicle of various stocks. Investing in a mutual fund is an excellent way to spread your risk since it pools together shares of stock from several companies. Unlike direct stock ownership, mutual fund investors own a portion of the fund. Additionally, fund managers at financial institutions often oversee mutual funds.
Machine learning approaches leverage the predictive capacity of a broad collection of mutual fund features easily accessible to investors. This ability allows investors to make more informed decisions about their investments.
How can machine learning be used to select mutual funds and stocks?
Algorithms that use machine learning may automatically learn the rules and predict market volatility by employing acquired historical data, which can help decision-makers make better choices. Machine learning can use large amounts of data and scrape the internet to construct complicated models that accurately forecast future market movements.
With this forecast, investors and fund managers can select what stocks to invest in by examining how various stocks will perform. With the aid of ML, investors may identify the factors that may affect stock prices before they begin to invest.
According to a study, researchers use a neural network to analyze various fund attributes to identify US mutual funds with the best performance. Their model predicts the top-performing decile of funds each month and calculates portfolio weights to maximize returns within that decile.
From 1980 to 2019, investing based on the model’s predictions resulted in a cumulative abnormal return of 72 percent. The findings suggest that investors can detect skilled managers and reallocate their investments accordingly. Additionally, fund inflows create buying pressure on the stocks held by funds, leading to higher prices and increased fund returns. This demand-driven momentum in fund returns contributes to the association between flows, fund momentum, and performance. However, the impact of changes in inflows takes time to materialize, and skill is reflected in the form of fund return momentum.
Overall, the study highlights the potential of machine learning techniques in predicting mutual fund performance and provides insights into the relationship between investor sentiment, fund flows, and fund momentum. Investors can exploit this knowledge to achieve higher returns by allocating their investments toward skilled managers and taking advantage of market dynamics.
The process of choosing stocks may also be automated with the help of machine learning algorithms. This may make it easier for investors to devote more effort to other parts of their portfolio management.
Also, when it comes to building a portfolio, machine learning algorithms may be used to tailor investment suggestions for investors by considering various factors such as risk tolerance, investment goals, and time horizon.
For example, machine learning in practice
Take the case of investment firm ABC, which places particular emphasis on the energy market. They just discovered a machine learning technology that can process massive volumes of data about energy providers. In this example, the resource draws attention to finding a sizable oil reservoir in an inaccessible area.
The machine learning model inside the application uses predictive analytics to foretell the oil industry’s profitability over a certain period and determine which energy equities are likely to appreciate in value. This investigation is carried out independently of the asset manager. This valuable data is then sent to the asset management so that appropriate action may be taken.
The asset manager may invest heavily in the firm’s shares based on the information provided by machine learning technology.
But let’s imagine things take a turn for the worst, and a big environmental disaster hurts the oil business, leading to a drop in stock prices. The machine learning algorithm modifies its settings automatically to account for the new data. It accomplishes this feat without requiring any alterations to the code by a human operator.
Because the asset management gets this further information quickly, they may act quickly to reduce losses by selling their shares in the afflicted energy firm.
Here, machine learning demonstrates its worth by giving asset managers access to relevant real-time data, allowing them to make more educated investment decisions and better respond to fluctuating market circumstances. Investment businesses may improve their portfolio management techniques and act swiftly in response to market fluctuations by employing algorithms and predictive analytics.
Tips on how to implement machine learning into mutual funds and selection of stocks
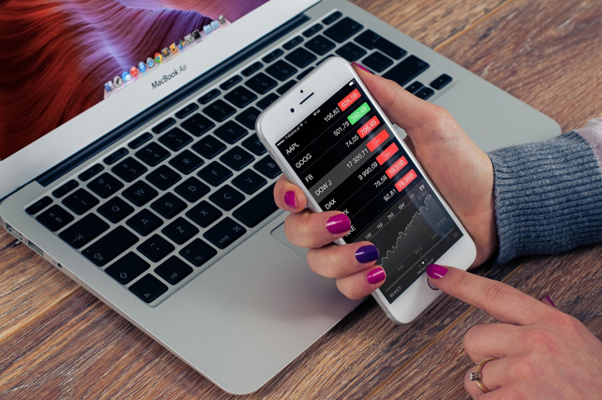
Image by Firmbee from Pixabay (Source)
When attempting to use machine learning (ML) to predict which investment option to pursue, adhering to a set of criteria is necessary. The following are some tips on how to include ML in the process of selecting stocks and mutual funds;
- Consider your investment goals and the resources at hand
The outstanding data analysis skills of ML are no substitute for the fact that the technology can only resolve some of the issues that investors face. Establishing precise needs and objectives by thoroughly assessing your current resources is a way to guarantee that machine learning can be successfully used in giving an accurate investment suggestion.
- Determine which machine learning methods are applicable.
Each of these algorithms has some benefits as well as drawbacks.
- Take into consideration the advice of a third party.
Developing and executing a solution based on machine learning (ML) takes a lot of work, particularly when considering implementing deep learning models. As a result, investors could decide to confer with ML specialists from a third party before getting started on their initiatives.
Benefits of using Machine learning to predict investments
- Reduction of emotional influence on investment decisions
Instead of taking calculated risks that may pay off, many investors make poor decisions that cost them money. There is no room for human irrationality in machine learning and algorithm trading.
- Algorithm trading
Investors need a massive quantity of information to make wise trading selections. However, investors are limited in how much information they can receive at once. An investor’s access to analyzing valuable market information may be improved via algorithmic trading.
By processing massive data, machine learning algorithms can provide more accurate and effective predictions.
- Increased investment opportunities
New investment possibilities may be found using a machine learning model because of its exceptional capacity to discover previously unknown connections.
Machine learning in mutual fund and stock selection has emerged as a transformative force in the investment landscape. Machine learning models can identify patterns, trends, and correlations that may not be readily apparent to human analysts, leading to improved portfolio performance and risk management. However, it is essential to recognize that machine learning is not a silver bullet and should be used with human expertise and judgment. While the technology continues to evolve and its applications expand, the integration of machine learning in investment strategies holds great promise for generating alpha and delivering value to investors in an increasingly complex and data-driven financial world.
Image by Markus Winkler from Pixabay (Free for commercial use)